Blog Post
Cyber Risk Quantification (CRQ) Models: How to Choose the Right One
April 11, 2024
“All models are wrong, but some are useful.” - George Box
In the earliest days of cyber risk management, chief information security officers (CISOs) generally relied on matrices and other subjective risk assessments for strategic planning. However, as it became apparent that this approach oversimplified the risk landscape, producing outcomes that were impractical for high-level communication, these industry professionals increasingly turned towards more data-driven methodologies, such as cyber risk quantification.
Cyber risk quantification (CRQ) is the process of determining, in numerical values, the likelihood an organization will experience a cyber event and the event’s respective financial impacts. When it's defined in these terms, CISOs and other cybersecurity leaders are empowered to assess cyber risk at the operational level and ensure it is thoroughly understood and managed according to key stakeholders' broader business objectives.
What is a Cyber Risk Quantification (CRQ) Model?
While CRQ, as a whole, encapsulates assigning numerical values to risk likelihoods and severities, there are multiple ways to conduct this type of evaluation. A cyber risk quantification model is a specific methodology or systematic approach that can generate these values. It provides organizations with a structured, repeatable, and defensible approach to assessing their cyber risk postures.
There are several models or approaches that cybersecurity experts leverage to quantify an organization's or enterprise’s cyber risk, including:
Expert Judgment Models
As the name suggests, expert judgment models and approaches to cyber risk quantification rely on the expertise of cybersecurity professionals and risk managers. These subject matter experts leverage their knowledge and experience to estimate the likelihood of their organizations falling victim to a cyber event and the potential financial impacts.
Although these cybersecurity leaders incorporate historical cyber risk data and current industry trends into their models, the results tend to be subjective and only defensible by the person who determines the data inputs. Within certain contexts, they can offer valuable insights that can assist with decision-making but should nevertheless be used with caution.
Deterministic Models
Deterministic models for CRQ are very helpful when assessing risks when all the relevant data is known, allowing risk managers to forecast outcomes with certainty. The deterministic approach is often used to create static risk scores based on factors that have been assigned an absolute value, such as the presence and maturity of security controls, vulnerabilities, and potential consequences.
While simple and relatively easy to understand, deterministic models unfortunately do not account for the complexity of the risk landscape or the market, both of which are highly volatile. Essentially, this methodology only evaluates the known knowns, but in cybersecurity, there are plenty of known unknowns, or uncertainties, that are necessary to factor in to achieve more accurate forecasts.
Probabilistic (Stochastic) Models
Stochastic models, also known as probabilistic models, harness statistical probability distributions, such as Monte Carlo simulations and Bayesian analyses, to produce a range of possible outcomes and scenarios an organization may face. This methodological approach to CRQ accounts for uncertainties and variability in cyber events, thus providing the information necessary for high-level strategization and initiative prioritization.
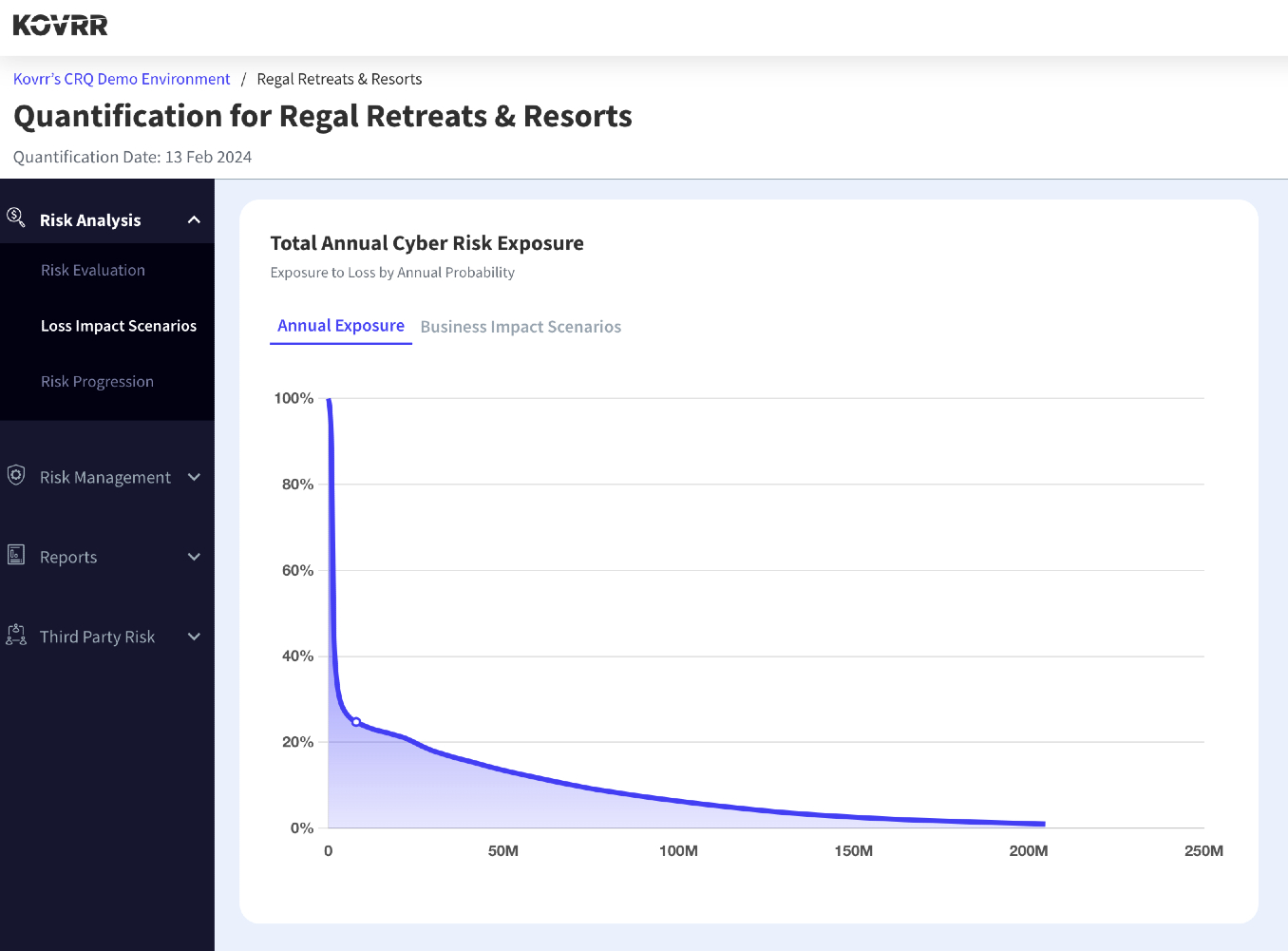
For example, in Figure 1, Regal Retreats and Resort's cyber exposure has been quantified using a probabilistic model. The model calculated that there is a roughly 80% chance of the company experiencing a cyber event or events that would result in a $1 million loss. At the same time, the organization has a 10% chance of suffering $30 million worth of damage due to a cyber event, illuminating the range of potential scenarios.
A Comparison of Two of the Leading CRQ Methodological Approaches
As CRQ has developed over the past few decades, several expert practitioners and third-party service providers have developed unique approaches to this quantification process, the majority harnessing varying combinations of the three models. Among these approaches, two have emerged to be the most prominent.
The Fair™ (Factor Analysis of Information Risk) Framework
The FAIR framework quantifies cyber risk by leveraging two key metrics: loss event frequency and loss event magnitude. Loss event frequency is calculated by considering how often a certain threat is likely to occur and the likelihood that the threat will result in financial losses. The frequency is then multiplied by the event magnitude, determined by primary and secondary financial losses.
Cybersecurity leaders manually gather all of this information by conducting research, speaking to key stakeholders, and making calibrated assumptions. These data points are then input into FAIR's mathematical algorithm, which includes probabilistic Monte Carlo simulations, to produce a range of cyber risks that an organization faces.

Conducting a FAIR framework risk assessment has become fairly commonplace, with approximately 50% of the US Fortune 1000 companies using it in some form or another, among many other corporate entities. However, despite its popularity, this approach has a number of drawbacks, such as its resource-intensive and subjective nature. These issues have emerged as especially precarious, considering how quickly the cyber risk landscape continues to evolve.
The Specific Challenges With the FAIR Framework
A FAIR framework analysis is highly resource-intensive, requiring cyber risk managers to gather all necessary information manually, which can pose major problems for certain organizations. Because data is collected by hand, it can take a very long time to complete the assessment. By the time results are rendered, the risk landscape or organizational structure may have been updated.
Moreover, the significant time investment makes the FAIR methodology impractical and uneconomical for scaling. Nowadays, board members and other high-level executives are interested in knowing the more granular aspects of cyber risks, such as vulnerabilities per department or other business unit. However, when it takes too long to conduct a single assessment, this information becomes too costly to gather.
The other primary challenge of the FAIR framework is also a result of the manual data-gathering process. As opposed to relying on objective, external sources and direct integrations, the subjective nature of that data input approach renders results unavoidably biased. Thus, the assessment is unreliable, potentially leaving organizations vulnerable to high-impact events.
On-Demand Cyber Risk Quantification Platforms
On-demand cyber risk modeling practices are similar to the FAIR framework in many ways but differ in other crucial areas. Indeed, most on-demand cyber risk quantification platforms, such as Kovrr's, leverage event frequencies and severity data to use in probabilistic models, subsequently producing a range of possible outcomes. On-demand solutions display this range as a loss exceedance curve, enabling high-level decision-making.
However, in stark contrast to FAIR, on-demand CRQ harnesses global loss intelligence data points gathered from external, objective sources. These data sources provide the necessary information regarding not only cyber events but also the cyber threat landscape, vulnerability and exploits, and business outages. Moreover, these on-demand CRQ platforms incorporate large-scale insurance loss intelligence to forecast financial damages based on real market losses.
In fact, because of this already-supplied data differential, on-demand CRQ modeling platforms offer CISOs a quick time-to-value. Quantified outcomes can be generated in roughly 24 to 48 hours, leaving cybersecurity departments free to pursue data-driven cyber risk mitigation programs that reduce an organization’s financial exposure, as opposed to wasting weeks devoted to manual data collection and research.
Moreover, as the name implies, the on-demand CRQ platform’s efficiency allows cybersecurity leaders to repeat quantification runs as needed. With CISOs now reporting to the board on a quarterly basis, this repeatability in a timely manner is especially valuable. Moreover, this advantage enables CISOs to test multiple scenarios rapidly and identify the variables that contribute most towards minimizing losses.
Finally, rather than relying on respective cybersecurity leaders to gather internal data regarding their organizations, on-demand CRQ solutions can integrate directly with connected systems, such as GRC, SOAR, EDR, BAS, SMDB, and IPS programs. This integration process inherently reduces the subjectivity of manual data entry, ensuring informational consistency and, therefore, higher result accuracy.
With the basis of projected cyber risk outcomes rooted in objectivity, combined with how fast organizations can access these outcomes, the on-demand CRQ modeling approach has emerged over the past decade as a distinctly attractive market solution.
Factors to Consider When Selecting a Cyber Risk Quantification Model
The optimal CRQ for an organization depends on multiple factors, the first of which is its size and complexity. In most cases, the larger a company is, the more intricate its infrastructure, systems, and communicating networks are, necessitating a more advanced CRQ model capable of investigating complex and interdependent risks.
Other internal business components that should be examined include the respective industry, cybersecurity framework, if any, and the relative maturity levels. These elements play a large role in forecasted event likelihoods and likely financial impacts. Lastly, being aware of risk appetite and tolerance levels is crucial, as some models will have more conservative risk estimates than others.
In addition to the internal business elements, CISOs must also carefully assess the CRQ models’ specific capabilities. For instance, what types of risks can the model capture, and to what extent can users explore the various factors that contribute to that risk? Scalability, time-to-value, and available integrations should also be considered in the decision-making process.
The Best Practices for Implementing a New CRQ Approach
Regardless of which CRQ methodological approach is adopted, the implementation process follows a relatively similar set of steps.
- Achieve Stakeholder Buy-In: Collaborate with key business stakeholders and executives throughout the organization, communicating the benefits of CRQ and how it transforms cyber risk mitigation into a holistic, data-driven process.
- Collect Data and Identify Assets: After colleagues are onboard, it’s time to gather the necessary data, both external and internal. Map the system structure, explore where crown jewels are positioned, research historical trends, and evaluate third-party service providers. This collection can be done manually or automatically via integration.
- Analyze Quantified Results: Once you’ve gathered all the necessary data, it’s time to run a quantification according to your selected methodology. Learn your organization’s expected event frequency and financial exposure, and drill down to more granular details such as event type and initial attack vectors.
- Align Mitigation With Broader Strategy: While it may be tempting to prioritize the mitigation initiative that targets the most severe risk, it’s important to align cybersecurity programs with broader business goals. Initiatives should ultimately enable growth.
- Continuously Monitor and Refine: Organizational structures and objectives change, new tools are implemented, and external risk landscapes evolve. Therefore, cyber risk quantification is a continuous process and should be conducted at least once a quarter to ensure programs reflect the latest threat environment.
The Optimal CRQ Model: A Contextual Decision
Ultimately, there is no one-size-fits-all CRQ modeling approach. Each organization has unique needs, characteristics, resources, and available expertise, all of which should be considered when deciding upon the “best” cyber risk quantification methodology.
While expert judgment models have the potential to offer valuable insights, probabilistic models provide a broader outlook on the risk landscape and allow for high-level strategic planning. However, in a similar vein, the FAIR methodology, despite being widely used, does not have the advantage of objectiveness and quick time-to-value, contrary to the on-demand CRQ approach.
The paramount goal of cyber risk quantification is to provide the CISO and other key executives with the information necessary to create a data-driven cyber risk mitigation strategy. The “best” methodology should facilitate this achievement.
To learn more about the benefits of on-demand CRQ and the advantage of a model that incorporates external global intelligence and insurance loss data at scale, contact one of Kovrr’s cyber risk experts today or schedule a free demo.
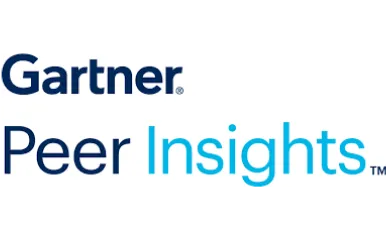

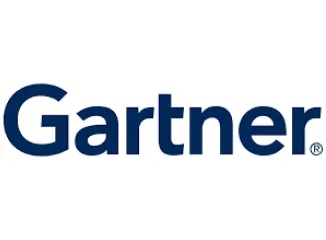