Blog Post
Moving Beyond the Cyber Risk Matrix With Defensible CRQ Data
December 11, 2023
The use of risk matrices for decision-making purposes extends back to the 1980s when the US Department of Defense needed a quick and easy way to evaluate hazards in safety systems engineering. Since then, the risk matrix has been applied in multiple fields to assess potential results and their associated risks, emerging as an instrumental tool for organizations grappling with making high-level strategic investments.
Using a Risk Matrix Model for Cybersecurity
The risk matrix's visual simplicity makes it highly accessible to associates at all levels of an organization, regardless of their particular field of expertise. Naturally, when cybersecurity evolved into a full-time career in the late 20th century, cyber professionals leveraged this historically helpful tool, utilizing it to foster a shared understanding of potential challenges and facilitate risk communication and resource allocation discussions.
While cyber risk matrices were undoubtedly valuable in the early days of cybersecurity assessment, the rapidly evolving risk landscape has now rendered them largely impractical, even damaging, when used in isolation. Due to their subjective nature and inherent functional limitations, cyber risk matrices cannot produce an assessment that accurately reveals an organization's most potentially likely and severe cyber events.
Modern cybersecurity demands more advanced approaches that incorporate internal and external global intelligence. As the digital threat environment continues to transform and cyber risk becomes increasingly frequent and severe, it's crucial to adopt a cyber risk quantification methodology that can produce unbiased, highly calibrated results, enabling executives to make data-driven decisions.
What Is a Cyber Risk Matrix?

A cybersecurity risk matrix is a grid that categorizes risks into different levels, typically ranking them as "low," "medium," or "high," according to a combination of their potential severity and likelihood. These subjective evaluations can also be assigned a numerical value, allowing for a pseudo-quantitative analysis.
These categorizations are also often color-coded, offering external evaluators an aesthetically pleasing and relatively straightforward overview of their organization's cyber risk. Because communicating with other executives who lack foundational cyber knowledge, Chief Information Security Officers (CISOs) find cyber risk matrices particularly helpful because of this advantage, using the results as a means to justify budget requests and initiative prioritization.
The Glitches in the Cyber Risk Matrix
Unfortunately, the risk matrix's simplistic nature, which allows for straightforward communication with non-technically oriented stakeholders, is paradoxically its downfall. In fact, the outcomes generated are hyper-simplified, which can lead to misguided resource allocation.
In his well-known 2008 article, "What's Wrong With Risk Matrices," Dr. Tony Cox discusses the many risks of the risk matrix, illustrating that using this inherently subjective approach potentially diverts attention away from the more severe and impactful risks, leaving organizations in a more vulnerable position than they were initially.
Nowadays, the numerous drawbacks to risk matrices are more than apparent, and cybersecurity teams should carefully evaluate their limitations before opting to use them.
Inherent Subjectivity of Cyber Risk Matrix Data
Cox's underlying argument situates itself on the premise that there is "no objective way to categorize severity ratings for events with uncertain consequences." Indeed, the levels in the risk matrix (called verbal risk labels) are not based on a standardized, scientifically justified framework that allows results to be externally verified.
Moreover, the definitions for these terms are, in actuality, just synonyms for the term itself. The subjectivity of the "low," "medium," and "high" classifications stems from the reliance on individual expertise or collective judgments as opposed to objective, quantifiable metrics.
Ultimately, these generalizations lack a concrete meaning. What one person may define as "high" another may consider "low," potentially rendering the results skewed.
Inconsistent Interpretations of Cybersecurity Risk Data
Because the results of a cyber risk matrix are inherently subjective, they are susceptible to interpretation biases among assessors. Different individuals or teams may perceive and assign risk levels differently, leading to inconsistencies in the evaluation process. The same set of variables might produce very distinct risk analyses.
At that point, the assessment becomes unreliable, as there's no way to measure accuracy. Additionally, the immense possibility for variability renders them non-transferable, leading to a sole dependency on the original assessor to evaluate results after security initiatives have been executed.
Compressed Range and Collapsed Uncertainty
By compressing both likelihood and severity into one-worded outcomes, or as Cox puts it, “lumping together very dissimilar risks,” cyber risk matrices completely disregard the underlying uncertainty that accompanies forecasting the future.
Cyber risks are more accurately expressed in ranges, such as a 40% to 55% chance of a data breach in the upcoming year. The rigid structures of matrices eliminate this granularity, framing risk in a falsely deterministic way.
Organizational cyber risk harbors numerous subtleties and nuances. Without examining the probabilistic nature of potential threats, CISOs and cybersecurity teams are left with an exceedingly narrow view of the risk landscape, leading to faulty mitigation plans.
Neglected Correlations
In the dynamic realm of cybersecurity, threats rarely operate in isolation, intertwining in intricate ways. However, matrices lack the analytical depth to make these connections, incorrectly segregating risks and resulting in an incomplete understanding of the environment. Failure to uncover these relationships may lead to unoptimized mitigation strategies that waste resources.
Moreover, even if each categorization is assigned a single numerical value (i.e., "high" is given a risk level of "5"), the risks are impossible to combine in a mathematically defensible way. Sure, you can perform math on the numbers, but the right math is dependent upon the relationship between the variables, of which there is none when expressed using ordinal values.
Would one add each of these risk levels together? Would it be better to calculate the average? As the number of risks continues to grow, the matrix's deterministic simplicity becomes a limitation.
Inability to Benchmark Against Peers
Peer benchmarking in cybersecurity is a valuable strategy for organizations to assess and improve their cyber risk posture. These comparisons offer insights into security effectiveness, help identify best practices, guide realistic goal setting, and ensure targets align with industry norms. However, risk matrices significantly hinder this ability.
The inherently subjective outcomes when using a cyber risk matrix render it challenging, if not impossible, for a cybersecurity team to assess its cyber programs against peers. Even if similar organizations used matrices that also measured cyber risk in terms of "low," "medium," and "high," there would be no way to gauge if these terms were commonly understood across company lines.
Furthermore, the broader investor community is provided very little additional information about your organization's cybersecurity resilience by knowing you have “medium” risk.
No Prioritization Roadmap
When all of an organization's potential risks are categorized into three or four different groups, the respective risks within each of those groups become impossible to prioritize. Without quantifiable criteria, there's no way to discern which of the "high" risks take precedence, as the matrix provides no inherent weighing system.
CISOs using matrices to evaluate their organization's cyber risk posture must eventually pursue mitigation initiatives at random, potentially leaving the system's most urgent vulnerabilities unpatched. While the "low," "medium," and "high" rankings offer some measure of hierarchy, the classifications do not provide enough to plan a robust, data-driven cybersecurity policy.
Absence of Actionable Insights
A cyber risk assessment should help CISOs make detailed, justifiable decisions. However, the data illuminated in a matrix does not assist in this endeavor. The results don’t offer any recommendations for what the cyber team can do to lower risk levels. The information gleaned provides a subjective overview of the organization’s risk landscape and little else.
The cyber risk matrix does not reveal, for example, which set of controls assists in mitigating a specific risk. Therefore, even once the cybersecurity team decides which project it will invest resources into next, there’s no data to verify that this project accomplishes the intended goal.
A Paradigm Shift: The Need for a More Precise, Data-Driven Approach
With Cox’s extensive research combined with increasingly misleading conclusions rendered from risk matrices over the past few decades, there is a growing appetite for a more structured, consistent framework that produces an objective overview of an organization’s risk landscape. As a result, CISOs have been turning towards cyber risk quantification (CRQ) models.
CRQ is the process of attributing economic values to a cyber event’s impact on an organization rather than classifying it according to arbitrary words. When these numerical values are transformed into event likelihood and financial consequences, the resulting insights enable CISOs to mature their cyber risk assessment and management.
Financial CRQ: Gaining the Necessary Insights for a Robust Cyber Program
Unlike the subjective nature of risk matrices, financial CRQ incorporates global cyber intelligence data, probabilities, and dependencies into rigorous statistical models to provide a more accurate and nuanced view of an organization’s risks.
As the risk landscape quickly evolves, harnessing data-driven CRQ tools is going to be critical in the fight against malicious actors.
Objective Data
Through quantitative metrics and measurable criteria, financial CRQ models can objectively evaluate the financial impact (severity) and likelihood (probability) of cyber events. This objectivity is essential as it ensures consistency, accuracy, and a reliable basis for decision-making that other external stakeholders can understand.
Consistent and Comparable
The objective nature of financial CRQ solutions enables organizations to assess risks uniformly. It ensures that, even if evaluated by different professionals, risk results will come out the same. Quantitative methods produce consistent, testable, and repeatable insights, which can then be used to compare risk outcomes with previous assessments or industry peers.
Clear Prioritization and Data-Driven Strategies
Event severity and likelihood provide a straightforward gauge of determining which risks are in the highest need of mitigation investments. When CISOs have this information, they can create justifying action plans and explain their prioritization decisions to the board.
In an age when there are too many cyber threats to combat simultaneously, prioritization is critical for safeguarding the most valuable assets. Similarly, such approaches provide defensibility when questioned by regulators and other stakeholders.
Granular Breakdown
Qualitative methods provide a detailed and specific view of risks, such as which business units house the most significant vulnerabilities and which type of attack an organization is most likely to suffer from. The granular breakdown is vital for understanding which controls and initiatives to invest in. For instance, if a financial CRQ reveals a high likelihood of a phishing attack, the CISO can focus on training sessions.
Precise Financial Information for ROI Determination
One of the most valuable aspects of CRQ tools is that they offer insights that facilitate financial planning. By understanding the monetary damages an organization should expect to face, CISOs and other executives can work together to develop a cyber risk appetite and ensure capital reserves are appropriately funded. Additionally, CRQ allows teams to evaluate whether initiatives will yield a positive ROI, a crucial detail if budgets tighten.
Red Pill or Blue Pill: Choosing a Cyber Risk Assessment That Accurately Reflects Reality
While this is by no means an exhaustive list of all the advantages financial CRQ solutions have in comparison to risk matrices, it nevertheless demonstrates that relying on antiquated grids results in ineffective, inaccurate cyber management practices. Incorporating on-demand quantitative models is not merely a strategically sound decision; it’s a necessity for remaining proactive in the ongoing battle against malicious actors and increasing regulatory scrutiny.
Before more advanced methodologies were developed, cyber risk matrices undeniably served as foundational tools for decision-makers. However, nowadays, as cyber threats continue to evolve, it’s critical for organizations to adopt quantitative models that guide data-driven planning and objectively-based collaboration to build high-end resilience programs.
Discover the limitations of these risk assessments and explore the shift towards financial CRQ models for more accurate, data-driven results that safeguard your most valuable assets.
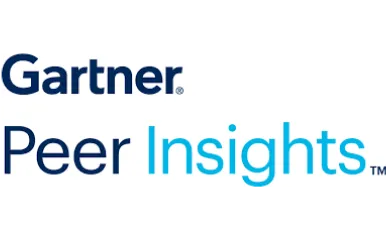

