Blog Post
The Value of Cyber Risk Quantification Models Vs. CRQ Frameworks
September 11, 2024
TL;DR
- Data-driven cyber risk management is essential to navigate the current threat landscape and proactively mitigate the potential impacts that accompany even the most non-malicious of incidents.
- While CRQ frameworks like FAIR can provide a structured methodology for analyzing an organization's risk, they lack the dynamic, real-time data necessary for accurate cyber risk forecasts.
- CRQ models, on the other hand, continuously consume and analyze vast amounts of cybersecurity data to provide crucial insights for more effective strategic planning and risk mitigation programs.
- Frameworks rely heavily on manual data collection, which is not only time-consuming and resource-intensive but often results in outdated threat information by the time the results are processed.
- CRQ frameworks like FAIR require specialized knowledge and training to understand and apply, making them less accessible and slower to implement than dynamic models.
- Kovrr's CRQ models, however, harness sophisticated probabilistic modeling techniques and extensive, continuously updating datasets to produce actionable insights that reflect an organization's latest cyber risk posture.
- Frameworks will remain insufficient unless enhanced by CRQ models, which ultimately ensure CISOs remain aware of emerging vulnerabilities and threats. Without this visibility, organizations remain vulnerable amid a cyber risk landscape that doesn't stop evolving.
Choosing Between a CRQ Model and Framework to Effectively Assess Risk
From the individual to the global level, managing risk is a part of life. While in some contexts, poor risk planning merely results in minor, inconsequential outcomes, in others, such negligence can be catastrophic. Take the July 2024 CrowdStrike incident, for instance, during which a faulty software update put global airlines out of commission, took broadcasters off the air, and cost the market upward of $5 billion in uninsured losses.
Amid today’s increasingly costly cyber threat landscape, adequate, data-driven risk management is crucial for businesses to remain not only afloat but wholly successful, a modern-day challenge that has compelled many chief information security officers (CISOs) to adopt various approaches to address. Within their toolkits, among other solutions, these cybersecurity leaders have access to both cyber risk quantification (CRQ) models and frameworks, which theoretically help them decipher this complex landscape and prepare.
However, if the underlying goal of the quantification is to gain a more comprehensive, accurate, and up-to-date understanding of the specific cyber risks their organizations face, then a mere framework will not suffice. Although they provide structured guidelines for assessing and managing risk, their value is severely limited without being supplemented by dynamic and continuously evolving CRQ models.
By discerning their differences, as well as learning how they overlap, CISOs will have no choice but to conclude that leveraging on-demand CRQ models is the only cyber risk management approach that will provide them with the data necessary to evaluate the specific risk drivers exposing their organization to cyber threats, and, therefore, help them to develop robust cybersecurity programs that achieve high-end resilience.
What Are Cyber Risk Quantification Models?
A model is a detailed yet simplified representation of a complex system or phenomenon that is fueled by data to help practitioners analyze them and, subsequently, forecast potential loss scenarios and their impacts. Some of the most common models are leveraged by professionals in the economic and environmental industries and used to predict specific market growth trends and climate changes, respectively.
In the context of CRQ, models operate similarly, continuously consuming and analyzing data related to historical cyber incidents, current threat intelligence, and vulnerability assessments to calculate the likelihood of various events occurring along with the respective financial impact. These models employ both statistical and probabilistic methods and provide real-time insights into a company’s cyber posture as it evolves.
A CRQ Model Example: Kovrr
Kovrr's on-demand CRQ platform leverages sophisticated probabilistic modeling techniques and incorporates extensive event frequency and severity datasets to produce a range of possible loss outcomes. By incorporating Monte Carlo simulations into the modeling process, Kovrr equips organizations with the knowledge that, for example, while they may face a 2% annual likelihood of experiencing a loss that exceeds $66 million, they face a much more likely, 40% chance, of these losses amounting to roughly $2 million.

With this range of information, informed by global loss intelligence data points continuously gathered from external, objective sources, Kovrr's CRQ models can likewise illuminate a myriad of core cyber risk metrics, such as Average Annual Loss, Average Events Likelihood, and respective peer benchmarking insights broken down according to industry and revenue bend. Kovrr’s models also highlight which specific cyber events and initial attack vectors contribute most to this financial exposure, aiding CISOs in their development of cybersecurity programs.
What Is a Cyber Risk Quantification Framework?
Frameworks, unlike models, are structured approaches that support the implementation or assessment of processes and systems, serving as foundations that organize the way various tasks are performed to ensure methodological consistency. They provide a comprehensive set of criteria, which can be adapted to various contexts and used in a multitude of fields, such as software development, project management, marketing, and education, to optimize workflow efficiency.
Illustrating the difference between models and frameworks using a simple analogy, such as a treasure hunt, can be particularly helpful. Within this context, a framework would be the treasure hunt’s rules, explaining the quest’s guidelines and ensuring everyone plays under the same set of parameters. Models, on the other hand, can be akin to the specialized tools that can make it easier to find the treasure, such as metal detectors. While operating within the framework's rule, these tools can offer direct data (a beep) in terms of where to find the treasure.
In the world of cyber risk quantification, a framework is a systematic methodology for analyzing an organization's unique cyber risk exposure, providing a set of principles and rules that cyber risk managers must follow to gain data-driven insights into the company's cybersecurity posture and identify the specific events it is most vulnerable to. Unlike CRQ models, however, frameworks are static, requiring regular updates to remain aligned with the current cyber risk landscape.
A CRQ Framework Example: The FAIR Standard
FAIR (Factor Analysis of Information Risk) is one of the most well-known cyber risk quantification frameworks; it is employed today by roughly 50% of Fortune 1000 companies to measure their respective cybersecurity postures. This common framework, first introduced in 2006, harnesses two metrics for the foundation of its approach to measuring exposure levels: loss event frequency and loss magnitude.
Loss event frequency is determined by how often a particular threat is likely to occur, coupled with the likelihood that it will lead to financial damage. This value is then multiplied by the loss magnitude, which is gauged by potential primary and secondary monetary losses. Cyber risk managers will gather all of this information manually and then input the final calculations into the FAIR mathematical algorithm that similarly includes probabilistic models to produce a range of possible loss scenarios.
While frameworks like FAIR can prove to be valuable for establishing a baseline understanding of an organization’s cyber risk, their reliance on manually amassed static data severely limits their effectiveness when used independently in a rapidly changing environment.
The Many Limitations of Cyber Risk Quantification Frameworks
Indeed, this manual data-gathering dependency is one of the primary reasons CRQ frameworks fall short of helping CISOs on a practical level. Amassing all this data is time-consuming, diverting cyber teams from mitigation efforts and, instead, compelling them to focus limited resources on data collection and analysis. By the time all of the necessary information has been compiled, the cyber risk landscape or organizational structure most likely will have already changed, rendering results obsolete.
Another major challenge of cyber risk quantification frameworks like FAIR is that they are incredibly niche and complex, requiring specialized training to understand the underlying quantification concepts, the process involved in the framework's unique approach to risk analysis, and how to interpret the results. The extensive time investment demanded even before implementation serves as a barrier to adoption and objective application.
Integration with existing risk management processes and systems is also a critical factor CISOs need to consider. CRQ frameworks are extremely limited in their integration capabilities, making it difficult to incorporate data from other platforms, such as SIEM solutions. This shortcoming likewise results in the absence of a unified source of truth, hindering data alignment and, therefore, reliable mitigation strategies. The risk of human error also increases, thereby compromising the risk assessment's objectivity.
The Value of Cyber Risk Quantification Models
Conversely, CRQ models continuously consume data from a wide variety of updated sources, providing CISOs with a cyber risk posture evaluation that reflects the latest external environment. The constant data influx enhances assessment accuracy, as it ensures that new threats and vulnerabilities are consistently taken into account. This real-time information is critical for the strategic prioritization of mitigation initiatives, significantly minimizing the likelihood that resources will be invested into non-critical issues.
Another key benefit of this automatic, real-time data consumption is that CRQ models have a quick time-to-value. Unlike frameworks such as FAIR, which require a considerable amount of resources to gather and assess internal cyber threat information, these models rapidly process vast amounts of data and subsequently generate actionable insights within a matter of hours. The combined speed and accuracy of CRQ models help CISOs respond swiftly to emerging risks, minimizing both their annual likelihood of occurrence and respective financial impacts.
Moreover, CRQ models excel in their integration capabilities, seamlessly incorporating data from various platforms, GRC systems, and cyber risk management tools to capture an organization's complete technographic profile. CRQ models can likewise quickly incorporate a company's chosen cybersecurity maturity framework (i.e., NIST, CIS, ISO) and respective implementation tiers into the quantification. This holistic integration reduces the risk of human errors and ensures that data is aligned and consistent across the organization.
By providing a unified source of truth, or singular hub for all cyber risk management information, CRQ models facilitate informed decision-making and support cybersecurity leaders as they develop programs that strive for resilience.
The Synergy Between CRQ Frameworks and Models
CRQ frameworks, when used in isolation to quantify cyber risk, are insufficient, as they lack the objective details necessary not only for accurate outputs but also for appropriate strategy development. However, when combined with a CRQ model that can account for all of the relevant data, frameworks can still offer a solid methodological approach that can be harnessed to produce an even more robust, comprehensive risk posture assessment.
For instance, in terms of generating a financial impact analysis of potential cyber incidents, a framework offers a calculation methodology, while the model can incorporate specific historical data and business-specific monetary information. Together, organizations can more easily understand the potential impact of events and allocate mitigation resources accordingly.
Returning to the metaphor of hunting for treasure, models are the tools, like compasses or metal detectors, that help the explorers find the loot in tangible ways. They ensure these explorers don't walk around in circles and instead take the most direct route to their goal. Frameworks, on the other hand, are the rules and guidelines that allow the explorers to justify their decisions and confirm that they were within their rights to take their respective actions. In cybersecurity, this is akin to demonstrating consistency and data reliability to the board.
By augmenting CRQ frameworks with CRQ models, CISOs can be confident that the cybersecurity programs they're developing reflect the current threat environment and can, therefore, prioritize initiatives according to the highest-impact risks. With such reliable information, achieving a state of cyber resiliency is not only possible but highly feasible.
Leveraging CRQ Models for More Effective Cyber Risk Management
As the costs of cyber incidents rise, new malicious actors emerge, and boardrooms continue to call CISOs into strategic meetings, relying solely on CRQ frameworks like FAIR is no longer sufficient for effective cyber risk management. While these frameworks provide a structured approach for assessing risk, they fall significantly short without the dynamic, data-driven insights that CRQ models offer.
With their ability to continuously consume and analyze vast amounts of cyber risk data on-demand, CRQ models ensure that organizations remain aware of new threats and vulnerabilities as they emerge, a non-negotiable capacity for strategic planning and effective mitigation prioritization. Ultimately, access to real-time data via CRQ models is not just beneficial but necessary. Without it, organizations risk falling behind in an ever-evolving threat landscape, leaving them vulnerable to large-scale consequences.
Staying Prepared and Resilient With Kovrr’s CRQ Models
Stay ahead of emerging threats and bolster your organization’s cyber resilience with Kovrr’s CRQ models, which consume millions of cybersecurity data points every day. Schedule a free demo or contact our cyber risk management experts today.
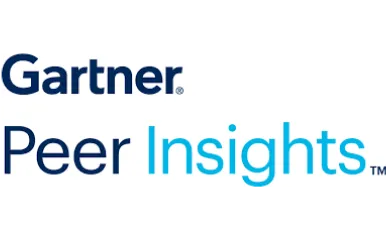

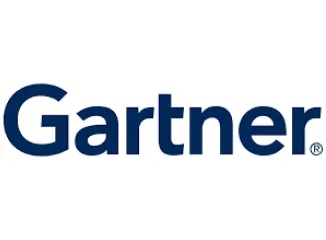